UNDERSTANDING HOW NDR CAN BE A POWERFUL ALLY
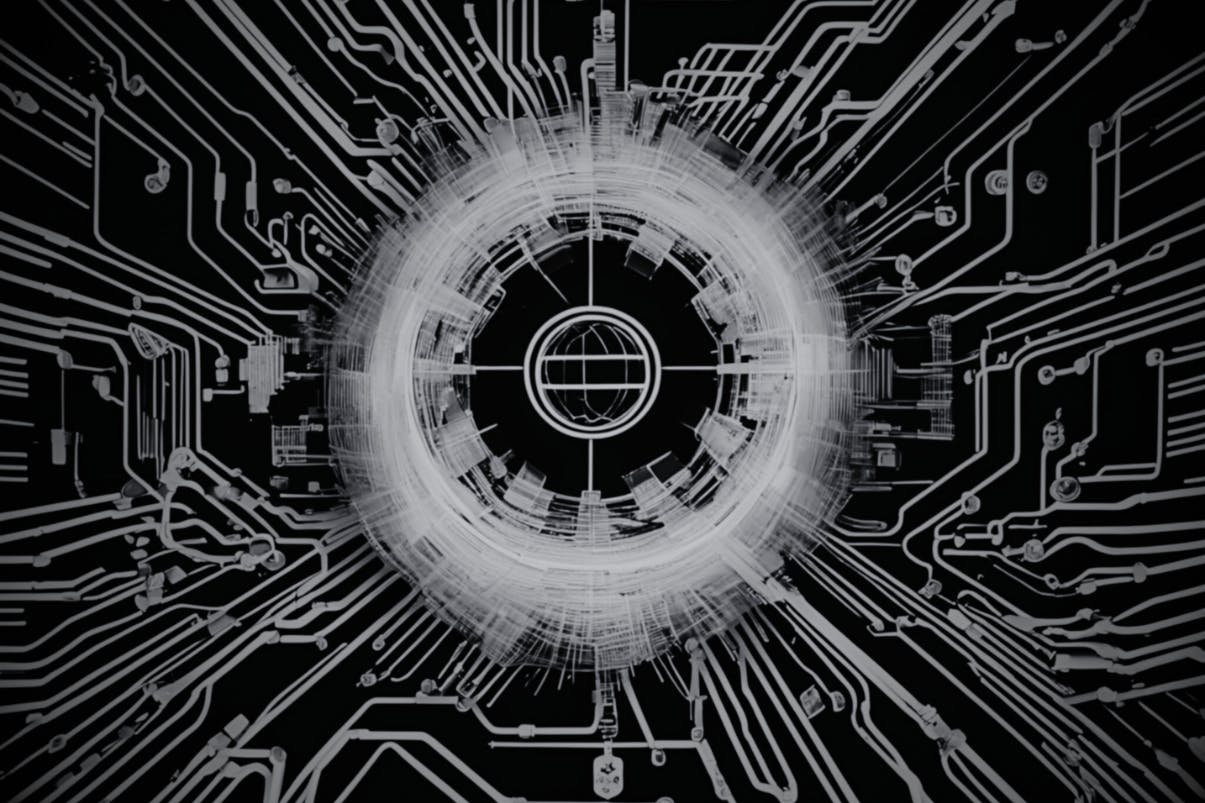
09 September 2024
NDR, Network Detection & Response, is a solution that allows real-time network traffic analysis and raises security alerts. It is a key element of any cyber defense strategy, as almost all attacks occur via the network. Therefore, detecting an attack upstream and allowing for reaction during the attack are some of the functionalities of this tool ; they are fundamental for protecting against cybercrime.
Its strength lies in the combination of different detection modules. Each module has its strengths but also its blind spots. It is this plurality of detection methods that elevates this tool several levels above each individual engine (such as an Intrusion Detection System or Network Traffic Analysis) and offers maximum protection coverage. It allows the detection of more complex threats, provides context to the various alerts, which are reliable, and subsequently facilitates threat hunting.
However, as with any tool, its effectiveness depends primarily on the user. That is why this article addresses different aspects of NDR, examples of detection engines that compose it, and how to use it to its full potential.
I - DETECTING WITH RULES USING NDR
a) THE IMPORTANCE OF SIGNATURES
One way of conducting detection is by using rules. These rules are mostly based on signatures, elements akin to indicators of compromise, which are markers of a cyberattack. Collected during past attacks, detecting these elements in one's network often indicates that an attack is underway.
However, it is not possible to detect all existing attack markers, firstly because there are too many for any detection tool to handle, and secondly because not all indicators are of equal interest. Indeed, an indicator is valuable only if it is likely to resurface and is significant enough that detecting it indicates an attack with a high degree of confidence.
An interesting concept was introduced in 2013 by David Bianco, named the 'Pyramid of Pain'. This pyramid ranks the different types of indicators or detectable techniques, but more importantly, the impact it has on the attacker, specifically how difficult it is for them to modify their attack method to avoid detection. At the base of the pyramid, we find virus hashes, which are very easily changeable for an attacker.
However, detecting them ensures an almost zero false-positive rate and also allows the detection of an attack conducted by a less advanced actor who would only reuse a malicious program they have obtained somewhere. It is important to place as many indicators in detection as possible because it forces the attackers to adapt and modify their tools with each attack. This protective measure is one of the most rudimentary and effective to implement and is a necessary effort to combat cybercrime.
To achieve this, a good understanding of the cyber threat landscape, the different attackers, malware, attack techniques, etc., is needed. This knowledge allows the identification, contextualization, and prioritization of the most critical signatures and thus the generation of the associated detection rules.
b) PATTERN DETECTION
In addition, it is possible to create detection rules that do not directly rely on signatures. For example, in the case of vulnerabilities, markers are visible and therefore detectable, such as requests containing certain specific elements or requests to access paths identified as related to exploiting the vulnerability.
One of the strengths of NDR is that it has an overview of network traffic. As a result, it can correlate, connect, and link different security alerts. But it can especially correlate different elements of the network traffic, which, independently, would not be considered malicious but, when put together, indicate an attack. For example, a failed SSH connection attempt is innocuous, but a hundred in a short period is a sign of an ongoing brute force attack. With an in-depth analysis of the attackers' modus operandi, it is possible to develop profiles and technical footprints of the attackers, which can then serve as a detection basis. For instance, if a well-known attacker typically uses IPs from the same autonomous system, downloads elements from these IPs, then performs a connectivity test by pinging Google's DNS, and finally uses the Telegram API, then each element alone is not sufficient to generate an alert. But if such activity is observed in this order concerning the same post, it is highly likely that it is a victim of a cyberattack by the identified attacker.
Thus, it is crucial for an NDR to have an effective, relevant, and limited set of rules to ensure a first line of defense against cyber threats.
II – HOW TO BETTER USE YOUR NETWORK DETECTION & RESPONSE
a) REGULARLY UPDATE YOUR SOLUTION WITH NEW RULES
Using rules is essential in a NDR solution. However, if the rules loaded on it are outdated or out of context, then they can become detrimental. Indeed, unnecessary rules pollute system performance, as each rule is evaluated for all traffic that passes through the NDR, but most importantly, they pose a problem to analyst for monitoring, as there is a high risk of generating false positives. Fortunately, with good processes behind it, these risks are avoided.
This is of paramount importance mainly for rules based on indicators of compromise. Although a hash - theoretically - cannot be the source of false positives, keeping signatures of malicious files that are no longer in circulation or used by threat actors in detection proves counterproductive in terms of performance.
In the case of IP addresses, they have a limited lifespan. It is rare for an attacker to use the same IP address for a long period, once their activity begins to be detected. Thus, either the service behind the address will be shut down, so no more activity is expected from this IP, or the attacker will discard the IP, and it will be reassigned to another user, without us being able to estimate whether that user will have legitimate activity or not. Even if this address is used by another attacker, we would have lost all contextualization around this address, and we could even mislead an analyst by leading them on a false trail. Moreover, some IP addresses are "multi-host," meaning this address hosts the services of many users, sometimes several thousand.
That is why it is necessary to constantly update your detection rule base, to remove indicators that have lost their value, but especially to add rules as quickly as possible related to ongoing attacks or malicious activities, as these are the ones that constitute the greatest risk. Similarly, if rules are used to detect attempts to exploit vulnerabilities, it may be wise to remove them once a security patch that fixes the vulnerability has been applied. However, we can decide to keep these rules if there are not already too many rules loaded, to monitor the network, and although the attempt will not succeed, detecting malicious activity is always interesting.
b) ADAPT YOUR RULES TO YOUR ENVIRONMENT
Another major aspect to improve your NDR's performance lies in adapting the rules to your specific environment. Most detection rules (including those produced by our Hoshi team), are intended to be general and are agnostic of the infrastructure. By knowing the equipment present in the environment, technological tools, nominal uses or behaviors, different IP address zones, or even specific IPs, it is possible to drastically improve the detection performance of the tool.
Indeed, some network activities may be entirely legitimate in a certain area of the network but absolute proof of a cyberattack in a different location. Similarly, an attacker's behavior using certain features, protocols, etc., once translated into detection rules, can be interesting if it does not correspond to a behavior already legitimately in place on the network. In this case, the rules would generate false positives, whereas these same rules can be valuable allies in a completely different environment.
That is why you should always adapt the selected rules and rule components to your environment and do this process iteratively, based on the number of alerts generated by these rules. This ensures that the rules are as refined as possible and subsequently simplifies the analysis of alerts, as they will be specific to the environment and provide more details.
c) DECRYPT TRAFFIC TO IMPROVE DETECTABLE FIELD OF VIEW
The NDR, through its multiple detection modules, allows for thorough network flow analysis and alert generation. However, it is limited by what it can observe. Nowadays, many communications are encrypted, and attackers use this to their advantage. They can communicate with their command and control servers via HTTPS, complicating the analysis and detection of these flows, or even use legitimate services such as Telegram or Google Drive.
There is a solution to partially solve this problem: SSL decryption. This involves setting up a series of proxies, which act as intermediaries between the user and the remote resource. An SSL-encrypted connection will be established between the user and the proxy, and then between the proxy and the remote resource. However, at the proxy level, the traffic can be decrypted and thus seen and analyzed by the engine. SSL decryption raises other issues, such as confidentiality, complexity of implementation, or hardware cost, but allows for much more extensive network traffic inspection and thus significantly improved detection.
III - HOW NDR CAN SERVE AS A DETECTION TOOL WITHOUT RELYING ON RULES
a) THE BEGINNINGS OF BEHAVIORAL ANALYSIS
The rule-based detection module is very efficient in its domain, but it does not easily detect new threats. This is why it must be accompanied, or coupled with, other modules. The NDR sees all network traffic and analyzes it, based on different metrics or methods that are not rule-based. This approach does not seek to detect past attack artifacts or elements known to be malicious but aims to identify anomalies in the network, abnormal behavior.
One of these analysis methods is entropy calculation. Entropy refers to a measure of disorder, or more specifically, in our case, the abnormality of a communication or network packet exchange, compared to communications considered normal or usual. Introduced by Yu GU, Andrew MCCALLUM, and Don TOWSLEY, this method follows the following process: the observed packets are classified into different similarity classes, based on various dimensions, depending on the number of properties to study (e.g., destination port, protocol…). It then establishes a probability distribution of a packet belonging to each class. The model is then established via maximum entropy estimation, first by selecting the most important properties and then weighting each property. Once the model is trained, it is enough to calculate the difference between the distribution of packets in the different classes and the previously obtained reference distribution. A significant difference indicates excessive "disorder" and, therefore, an anomaly in the network. This approach, although simplistic, already allows for anomaly detection in traffic, with minimal computing power.
A second analysis method, presented by Maciej SZMIT and Anna SZMIT, is the use of the Holt-Winters method applied to anomaly detection. The Holt-Winters model is an adaptive model that is relevant in an environment that shows periodicity, recurring behaviors, and trends. The goal is to identify behaviors that are different from what the model predicts based on previous cycles. The authors use a double periodicity, the first representing a day, and the second a week. It is then possible to detect anomalies using the brutlag algorithm. This method has the advantage of considering recurrence, habit, and temporality as the main elements of detection. Thus, a behavior that may be legitimate on a Monday because it is from a weekly task may be considered an anomaly if it occurs on a Wednesday because it does not occur at the right time in the cycle.
b) THE ADVENT OF ARTIFICIAL INTELLIGENCE
Behavioral analysis has been able to take on new momentum with the rapid progress of artificial intelligence. NDR is a tool that can also embed AI to significantly improve detection quality by adding yet another complementary layer.
Different concepts from various fields can be used, such as those from machine learning. Many algorithms or models have been tried, evaluated, and described in the scientific literature.
The first approach is the use of a genetic algorithm. This type of algorithm is an iterative approach that considers multiple properties of 'individuals' within a 'population.' At each step, a set of individuals is selected to generate the next set of elements, and naturally, individuals with the best properties survive and become increasingly predominant. In the context of anomaly detection, the properties are connection elements (IP addresses, number of bytes, protocol, etc.). The goal is to conduct a training phase to find a model that converges to detect only abnormal connections. Each training packet is analyzed, and if it is a deviant packet, then the individual's property set receives a bonus in the scoring system; if it is a normal packet, it receives a penalty. The population then evolves to find a set of conditions that will allow anomaly detection in the production environment once training is complete. One disadvantage of this solution is that it requires a lot of computational power.
A second approach is based on the principle of naive Bayesian classification. Traffic is analyzed, and based on past events, a probability is determined that an event will happen in the future. Despite the apparent simplicity of this method, several studies have shown that naive Bayesian classification can be more accurate and faster than other sophisticated methods, especially as the dimension of the input data increases.
Finally, a third elementary method of AI-based anomaly detection in an NDR is the use of decision trees. This well-known supervised learning method aims to establish a series of tests on different attributes to determine whether a packet is legitimate or not. It first requires a training phase in which the model will determine the classification criteria from a known dataset. Once this phase is complete, the model can then be used for detection. One of the advantages of decision trees is that it is easier for the model to explain its results and indicate which criteria it considers to determine whether an element is legitimate or not.
c) THE USE OF AI IN JIZÔ NDR: DEEP LEARNING
Jizô's proprietary AI is based on fundamentally different concepts. No learning is supervised. The model is not trained on malware or known attack databases. Its goal is to detect what is not normal or usual. The major advantage is that it can detect all types of attacks, known and unknown, through network traffic analysis. This is complemented by a second aspect, which is behavioral analysis not on the network as a whole but for each actor present in the network.
This profiling is an important element that allows us to know whether an activity is usual or not for each individual.
Finally, the generative AI integrated into Jizô provides assistance not directly for pure detection, but helps the analyst to better understand the technical information related to detections. It also provides leads and recommendations on possible courses of action when an alert indicates an abnormal situation. By playing both on the technical and human fields, the AI embedded in Jizô offers a new dimension to detection via NDR.
Adversarial Learning
One of the dangers associated with using artificial intelligence in threat detection is adversarial learning. This method, used by attackers, aims to gradually inject infinitesimal modifications into regular traffic, so that the model learns from these modifications and no longer identifies this type of communication as abnormal in the future. Although interesting, this approach by attackers could not disrupt Jizô, because for it to have a real influence on the model and its detection capacity, it would require a very large volume of traffic. However, this traffic coming from the adversary will be quickly detected and thus the attempt to manipulate our AI revealed.
Behavioral detection is a fundamental prerequisite to complement rule-based detection and other modules, mainly for detecting unknown threats.
FOR OPTIMAL DETECTION, NDR MUST BE COMBINED WITH OTHER SECURITY TOOLS
The NDR is a complex tool that offers many possibilities for intrusion detection. For optimal detection, its strengths must be combined and different detection methods used to cover the blind spots of other methods or to reinforce their decisions. It is this cooperation, this teamwork between the modules that makes it indispensable. However, the usefulness of this tool also depends on the environment in which it is placed, its adaptation to it, how it is supervised, and what actions are taken for each security alert. The NDR is an indispensable tool in any security architecture and must interface with other tools (EDR, SIEM, etc.) to offer maximum detection capacity.
Jizô, the observability platform qualified by ANSSI, has the advantage of innovatively combining all these forms of detection to offer the best possible protection. Thanks to its various integrated engines, it is an indispensable tool for identifying, anticipating, and blocking cyber threats. It notably includes signature-based detection, supported by a dedicated cyber threat intelligence team, as well as heuristic detection. Artificial intelligence is also at the heart of the solution, with very advanced algorithms from unsupervised continuous learning deep learning. In addition, Jizô handles both the IT world and the OT world, with an innovative connection between the two worlds. The real strength of Jizô is that its value goes far beyond the sum of the independent values of each detection engine. It is the cooperation between all these that allows for unmatched detection power.
Finally, Jizô has many interconnection interfaces with other security equipment, such as Security Orchestration, Automation and Response (SOAR) or Security Information and Event Management (SIEM), allowing it to perfectly integrate into any cybersecurity architecture.